
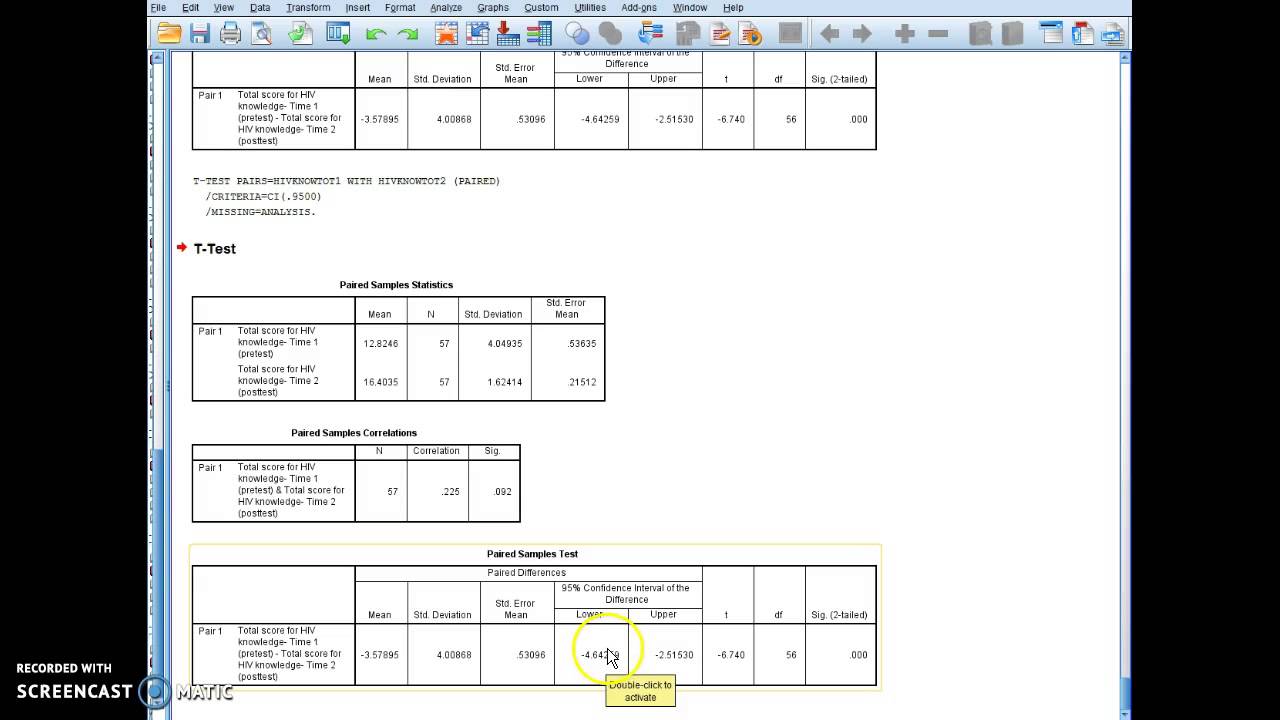
For example, there must be different participants in each group with no participant being in more than one group.
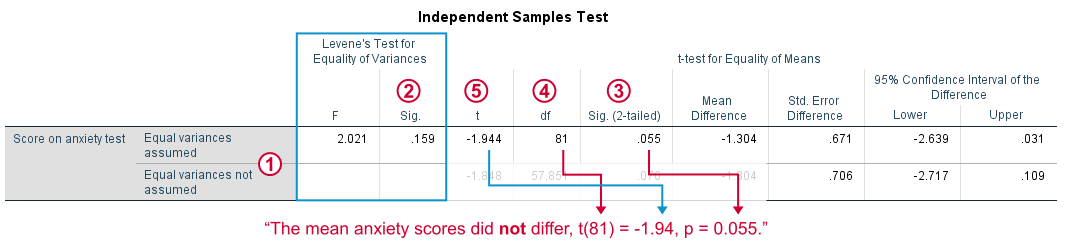
Example independent variables that meet this criterion include gender (2 groups: male or female), employment status (2 groups: employed or unemployed), smoker (2 groups: yes or no), and so forth.Īssumption #3: You should have independence of observations, which means that there is no relationship between the observations in each group or between the groups themselves. Examples of variables that meet this criterion include revision time (measured in hours), intelligence (measured using IQ score), exam performance (measured from 0 to 100), weight (measured in kg), and so forth.Īssumption #2: Your independent variable should consist of two categorical, independent groups. First, let’s take a look at these six assumptions:Īssumption #1: Your dependent variable should be measured on a continuous scale (i.e., it is measured at the interval or ratio level). Even when your data fails certain assumptions, there is often a solution to overcome this.
T test spss how to#
This is not uncommon when working with real-world data rather than textbook examples, which often only show you how to carry out an independent t-test when everything goes well! However, don’t worry. In practice, checking for these six assumptions just adds a little bit more time to your analysis, requiring you to click a few more buttons in SPSS when performing your analysis, as well as think a little bit more about your data, but it is not a difficult task.Before we introduce you to these six assumptions, do not be surprised if, when analysing your own data using SPSS, one or more of these assumptions is violated (i.e., is not met). You need to do this because it is only appropriate to use an independent t-test if your data “passes” six assumptions that are required for an independent t-test to give you a valid result. For situations when the populations have different shapes, larger samples are needed.When you choose to analyse your data using an independent t-test, part of the process involves checking to make sure that the data you want to analyse can actually be analysed using an independent t-test. The t procedure for "Equal variances not assumed" is particularly robust when the sizes of the two samples are equal and the distributions of the two populations have similar shape, even when sample sizes are small.The "Equal variances NOT assumed" is the generally preferred method, given the difficulty of assuring equal variances. The results for "Equal variances assumed" uses the pooled t test method, which has exactly a t distribution. The analysis results for "Equal variances not assumed" uses the general t test method, which approximates a t distribution.The image below displays a portion of the output for a t test of SAT Math scores comparing male and female computer science students. The independent samples t test is fully specified. The Independent-Samples T Test Options dialog displays.
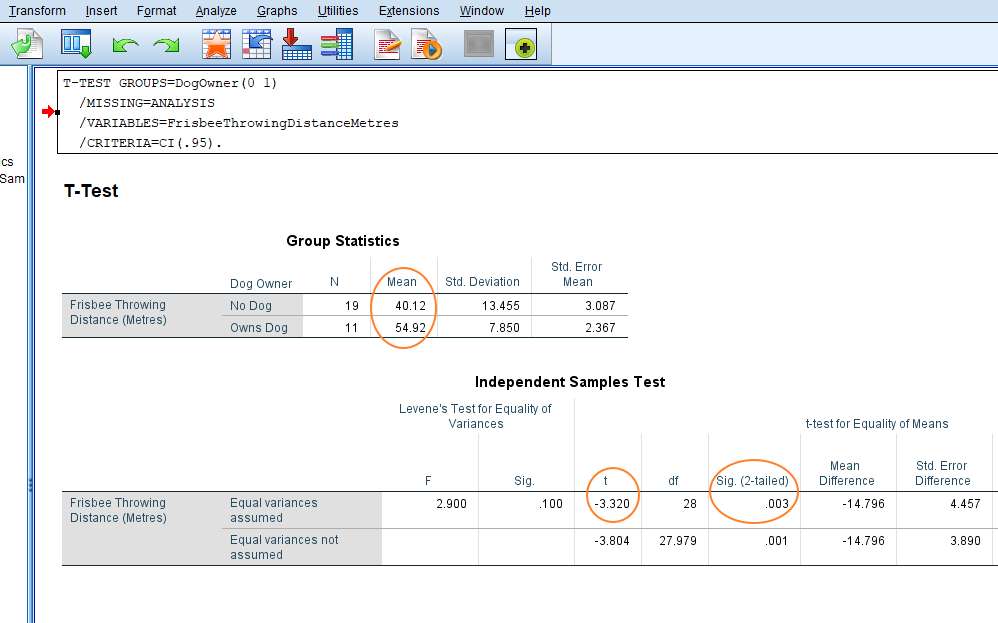
Note that the difference in means is calculated as Group 1 - Group 2. (For example, if the variable Sex in the dataset is coded 1 (meaning male) and 2 (meaning female), entering a 1 for Group 1 indicates that Group 1 is the male group. The Group 1 and Group 2 values are the data values for the grouping variable that indicates membership in group 1 or group 2. button under the Grouping Variable window. Select the categorical (measurement level=nominal or ordinal) explanatory variable and use the arrow button to move it to the Grouping Variable window.Select the quantitative (measurement level=scale) response variable and use the arrow button to move it to the Test Variable(s) window.The Independent-Sample T Test dialog displays. Select Analyze > Compare Means > Independent-Samples T Test.The number of rows should be equal to the total number of observations in the study (i.e., n 1+n 2). The grouping variable can be numeric or string (e.g., 1 or 2, A or B, male or female), although beware of included spaces if variable set as string. For two independent samples, we need at least two columns in the dataset: one column to contain a grouping variable (the explanatory variable) and another column to contain the test variable that will be compared (the response variable).
